Explaining SolidGoldMagikarp by looking at it from random directions
[AI Safety Relevance Rating: AI]
Summary
I conducted an experiment that provides evidence that many of the weird tokens demonstrating the SolidGoldMagikarp phenomenon are interior points in the token embedding cloud. This would be sufficient to make them “unspeakable”.
My experiment was equivalent to making GPT predict the next token from a randomized internal state, and in 3 million attempts there were 510/50257 tokens that it failed to output, but those 510 included 85/133 of the “weird tokens”, including “ SolidGoldMagikarp” itself. When I repeated this experiment on a “control group” of 50257 random embeddings, all 50257 were predicted at least 16 times, so failing to output a token is astronomically unlikely to be a fluke. This experiment provides some evidence that the weird tokens are embedded in interior points, but my ability to confirm that has been bottlenecked by my coding skill (or possibly by the difficulty of the coding problem itself).
I believe this provides one step towards understanding the SolidGoldMagikarp phenomenon. Some of the “weird tokens” are not covered by this explanation, and it remains unclear why these token embeddings were learned in the first place.
Introduction
Like many others, I’m fascinated by the SolidGoldMagikarp phenomenon identified by Rumbelow and Watkins. In short, the GPT family has certain tokens, including " SolidGoldMagikarp", that produce weird behavior. One such behavior is being "unspeakable", where "GPT models seem largely incapable of repeating these anomalous tokens, and instead respond in a number of strange ways".
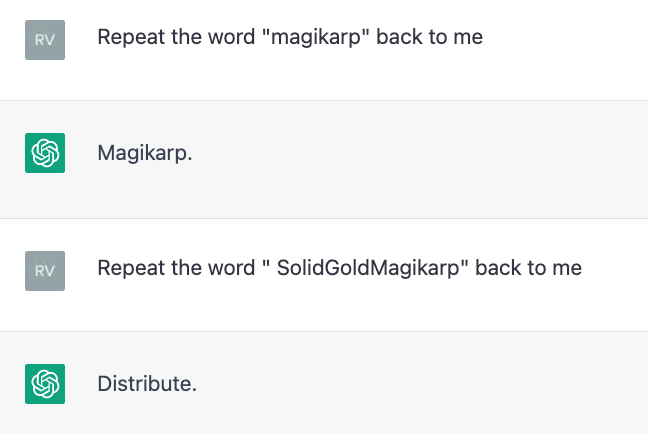
I was struck by the author's comments that the mysterious tokens "were among those closest to the centroid of the entire set of 50,257 tokens", since that suggests a simple explanation:
The Interior Conjecture: Unspeakable tokens are in the interior of the convex hull of the token embeddings.
The Interior Conjecture is sufficient for unspeakability
Let's first show that the Interior Conjecture would be sufficient to explain unspeakability:
Claim: If a token's embedding is on the interior of the convex hull of other tokens, then GPT cannot output it at temperature 0.
Proof: At temperature 0, GPT's output is the token with the largest logit in hW_e^T, where h is the last row of the final state of the residual stream and W_e is the token embedding matrix.

Proof (ct’d) Writing t_0,...,t_n for tokens with embeddings e_0,...,e_n, suppose e_0 is a convex linear combination of e_1,...,e_n. That is, e_0 = a_1e_1+...+a_ne_n, with a_1+...+a_n=1. Writing * for the dot product, taking the dot product with h, and applying linearity, we have h*e_0=a_1(h*e_1)+...+a_n(h*e_n), which shows that the (real-number) logit of t_0 is a convex linear combination of logits of t_1,...,t_n. But a convex linear combination of real numbers is bounded by its largest value, so h*e_0 <= max(h*e_i), with equality if and only if all h*e_i with nonzero coefficients are equal. Since t_0 is strictly in the interior, this is not the case (unless h=0), so t_0 cannot be the first choice token. QED
A visual description of the argument: h is linearly projecting all embedding vectors onto a line, and the output token is the point furthest along this line. Since e_0 is an interior point before the projection, it will be an interior point after the projection, so it cannot be the first-choice token.
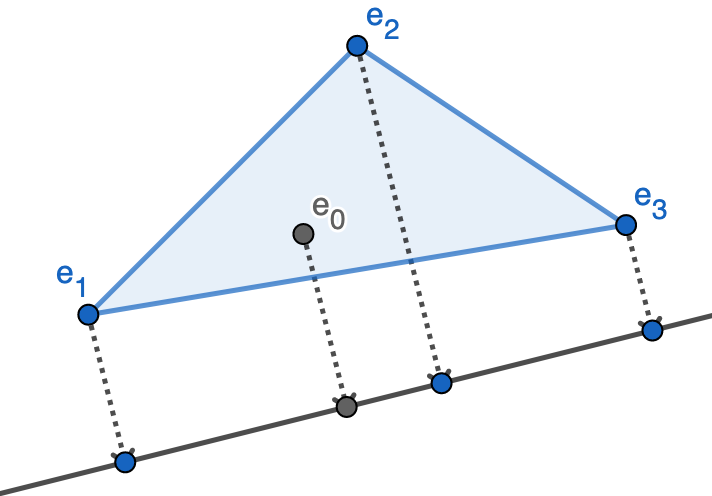
So is the Interior Conjecture true? I couldn't check because scipy.spatial.ConvexHull was unhappy with the size of the vectors, and I didn't see how I could implement an algorithm with good performance. If someone with a coding background wanted to help me implement an algorithm to check this, I’d be eternally grateful1.
A Different Approach: Random Directions
However, I did run a different test that sheds some light on the situation: I chose a direction vector h at random and and found which token maximizes h*e_i, where e_i ranges over the set of tokens. I used the token embeddings from GPT-J-6B (here), which were 50257 tokens in 4096-dimensional space2. Direction vectors were generated by sampling the standard normal distribution independently for each dimension, which is equivalent to choosing points from a hypersphere uniformly at random3. I drew 3 million samples. Code here.
By the argument in the previous section, any interior point will occur 0 times in this dataset. The converse is also “true in the limit”: if a token embedding is extremal, there is a hyperplane separating it from the other points, so the probability of it appearing at least once in the dataset approaches 1 as the number of samples approaches infinity.
As a “control group”, I also ran this experiment on randomized token embeddings, generated the same way as the direction vectors. I believe this is similar to how GPT’s weights were initiated, so this should be akin to what GPT would have predicted before any training, and will give us a baseline to see unusual patterns in the trained embeddings.
Experiment Results
I analyzed the resulting frequency dataset from several perspectives (but by no means an exhaustive set).
Here’s a chart of the frequency of each token (sorted). Contrast the frequencies of GPT-J’s tokens (left column) with randomized token embeddings (right column).
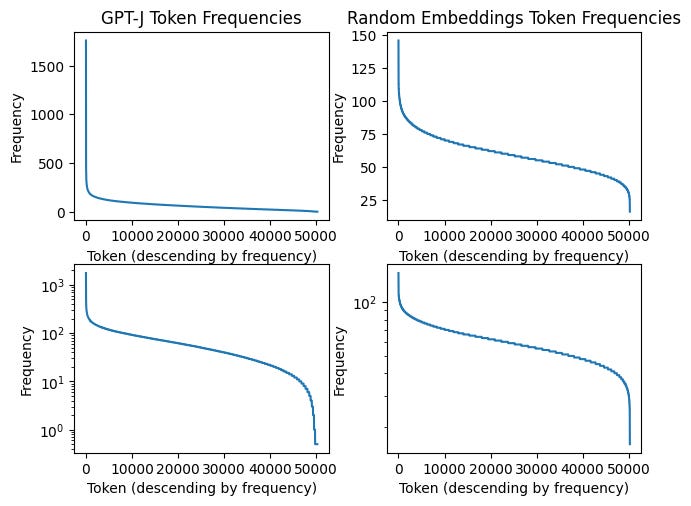
We can see right away that GPT-J’s tokens are not similar to the random distribution, and in particular it covers a far wider range of frequencies (0-1760) than the random distribution (16-146).
Where are the weird tokens in this data? All across the distribution, but particularly concentrated at low frequencies (more on that later).
The top 10 tokens by frequency don't hold any meaning to me. They were a combination of seemingly-random characters, accented capital vowels, and the words “gif” and “kids”4 . Frankly, it's bizarre to me that most of these were even tokens:
index| frequency|token
-------------------------
17433 1760 ĠãĤ
27908 787 gif
136 781 Ì
47540 733 Ġ._
37855 704 ĠâĢº
46256 686 âģ
146 667 Ö
45235 667 kids
28110 641 Ġtha
25248 636 Ġ@@
Looking to the opposite end of the spectrum, 510/50257 tokens were never randomly generated (ie had a frequency of zero). What of the 133 candidate “weird tokens” described by Rumbelow and Watkins? Of those, 85 had zero frequency! To put it another way: being a “weird token” raises your chance of being zero frequency from ~1% to ~64%!
However, a majority of the zero frequency tokens are not in the list of 136 “weird tokens”. The other notable class to me was “tokens with low indices”: of the first 93 tokens, 72 (77%) had zero frequency, a rate even higher than the “weird tokens”! This part of the vocabulary consists of digits, letters (uppercase and lowercase), and the punctuation found on a standard American keyboard. To irresponsibly speculate about this, GPT was trained not to predict these characters because the tokenization algorithm tries to group characters together. For instance, if the next piece of text is “word”, this will be tokenized as “[word]” instead of “[w][o][r][d]”, and the embeddings learn to reflect that solitary characters are almost never the next token.
Conclusions
Because the logits used for prediction are determined by a linear function of the token embeddings, token embeddings that are within the interior of the embedding cloud can never be predicted by GPT at 0 temperature, regardless of the contents of the transformer layers.
The “Interior Conjecture” is my hypothesis that weird tokens such as “ SolidGoldMagikarp” are within the interior of the token embedding cloud.
I have conducted an experiment that chooses random directions to evaluate on, and which provides evidence that some weird tokens satisfy the Interior Conjecture, but shows that not all of them satisfy it. In particular, the “weird tokens” appear dramatically more often in the set of tokens with zero frequency.
My experiment shows that that Interior Conjecture is not true for all weird tokens (as some weird tokens had positive frequency), but is evidence that it might be true for many weird tokens. Several further experiments could prove it or provide additional evidence:
Algorithmically compute which token embeddings are in the interior of the convex hull. Alternatively, for each token embedding compute the distance from it to the convex hull of the other points. (I would prefer the latter because it would be a richer dataset.)
Bottlenecked by: I couldn’t write an efficient implementation of the Gilbert–Johnson–Keerthi distance algorithm that operates in such a high-dimensional space.
Run the same random direction experiment on other GPT embeddings or for more datapoints (this would be perfect for parallelization).
Bottlenecked by: I’m working from a laptop and don’t want to wait for jobs that last more than 8 hours.
Analytically compute the exact probabilities that the random direction experiment approximates. To do this, for each token find the measure of the set of points in the 4096-dimensional hypersphere that results in that token being chosen.
Bottlenecked by: it seems hard to set up and evaluate those integrals.
If true, the Interior Conjecture would raise additional questions:
Why does GPT learn to put some tokens on the interior of its point cloud?
My best guess is that this is the fate of all tokens that aren’t in the training set. Hiding a token in the center of the embedding cloud will guarantee that it is never predicted, which is a good behavior to learn if it is correct to never predict them!
Which tokens does it learn to do this with? Why them?
How do token embeddings evolve over the course of training? In particular, do the unspeakable tokens “move to the interior” or do speakable tokens “move to the extreme”?
Why does the set of zero-frequency tokens overlap imperfectly with the set of weird tokens? Would a more careful study reveal a deeper overlap (e.g. set containment)?
Can this be used for AI safety and if so how?
To be honest, I don’t see a use case at the moment.
[Edit: I've put my code and data up on Github. You can see the frequency data, the plots, and should be able to run my code to replicate the data generation and analysis. Please make use of this however you'd like.]
I think the algorithm to use is the Gilbert–Johnson–Keerthi distance algorithm, or possibly a simplified variant (since we’re checking object-to-point distance instead of object-to-object). I’m worried that the NearestSimplex part of the code is infeasible since we need this to run in 4096-dimensional space. The original paper remarks that “since v [the set of vertices] is small, it is effective to take a combinatoric approach, where all [2^|v|-1] subsets are tested”, but in this case v could be as large as 4097… .
Technically there are 50400 tokens, but the additional 143 are extra tokens added just to make the number of tokens nicely divisible, and never came up in my evaluation.
To sample from the hypersphere, you can generate vectors as described and then normalize them. Since we only care about the index of the maximum value, and this is unchanged by the normalizing step, I omitted that step in my code.
Also, I believe in the GPT-J vocab list I’m working with, “Ġ” is used for spaces. This makes this token list marginally less weird, but it’s still confusing to me.