Rating my AI Predictions
9 months ago I predicted trends I expected to see in AI over the course of 2023. With the year coming to a close, let’s rate how I did!
Predictions
ChatGPT (or successor product from OpenAI) will have image-generating capabilities incorporated by end of 2023
My prediction: 70%. Resolution: Correct
No papers or press releases from OpenAI/Deepmind/Microsoft about incorporating video parsing or generation into production-ready LLMs through end of 2023
My prediction: 90%. Resolution: Correct
We had a bit of a wobble there with the Gemini trailer, but since then Google has confirmed they “used still images and fed text prompts” to make that trailer. So, I’m going to count Gemini as not incorporating video parsing into a “production-ready” LLM. I also did a search for progress I might have missed, finding some attempts like this, but none of it has reached the “production-ready” level yet.
All publicly released LLM models accepting audio input by the end of 2023 use audio-to-text-to-matrices (e.g. transcribe the audio before passing it into the LLM as text) (conditional on the method being identifiable)
My prediction: 90%. Resolution: Incorrect
Here’s a video of a Deepmind research scientist explaining that Gemini directly embeds audio, and does NOT simply transcribe.
This prediction feels like a big blunder in retrospect. I think my thought process was:
Transcription is easy.
Direct embedding is harder.
(1)+(2) imply no one will do the hard thing of direct embedding.
But in retrospect 1 and 2 don’t imply 3. This mistake feels especially obvious in retrospect since I otherwise predicted models would grow into other modalities, so I should have allowed at least some room for pushing out the frontiers of audio.
All publicly released LLM models accepting image input by the end of 2023 use image-to-matrices (e.g. embed the image directly in contrast to taking the image caption) (conditional on the method being identifiable)
My prediction: 70%. Resolution: Correct
GPT-4 accepts images via GPT-4V, whose system card says “[to train GPT-4V t]he pre-trained model was first trained to predict the next word in a document, using a large dataset of text and image data”. Microsoft’s Copilot (previously Bing chat) lets you “copy, drag and drop, or upload images to chat”, and I’m not sure if they ever explained their training process but since they collaborate with OpenAI I assume its the same.
Google’s Gemini dumps everything into a transformer, as predicted:
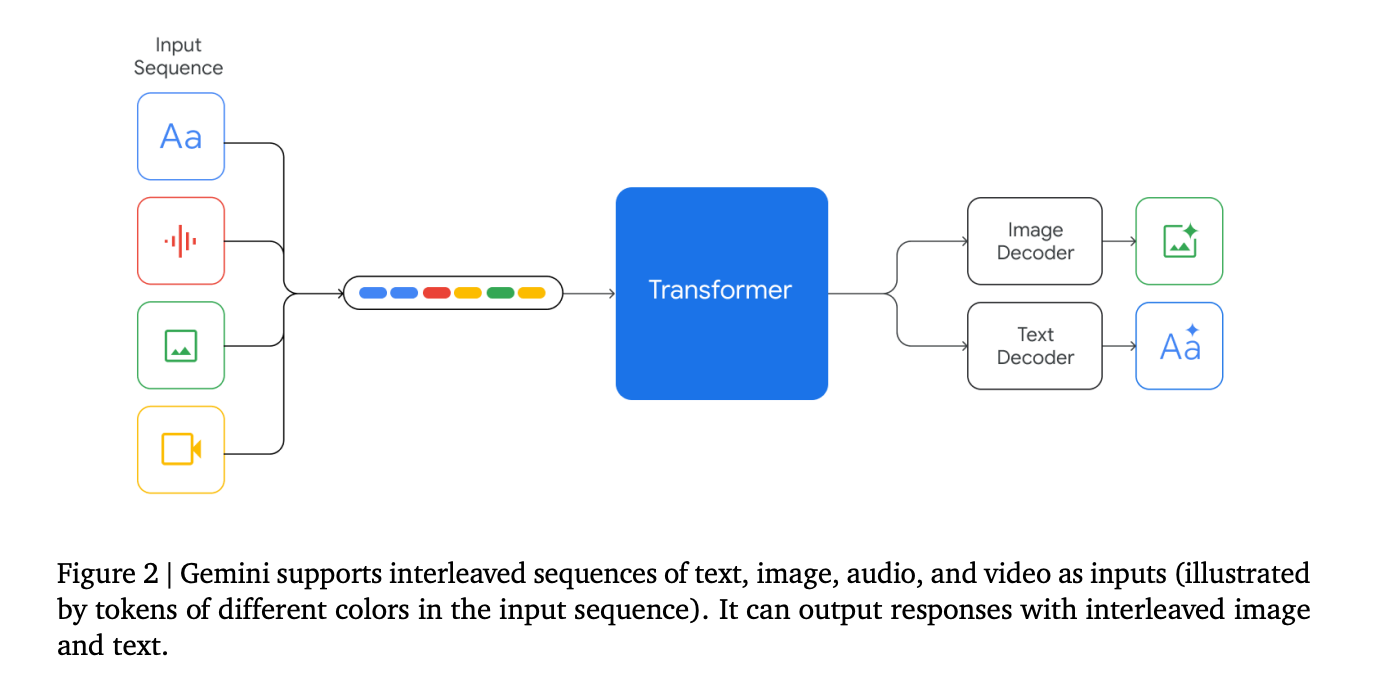
If I’m missing others, let me know.
At least one publicly-available LLM incorporates at least one Quick Query tool by end of 2023 (public release of Bing chat would resolve this as true)
My prediction: 95%. Resolution: Correct
This came true blindingly fast, but here’s a simple example of ChatGPT doing this for weather:
ChatGPT (or successor product from OpenAI) will use use at least one Quick Query tool by end of 2023
My prediction: 70%. Resolution: Correct (see previous image)
The time between the first public release of an LLM-based AI that uses tools, and one that is allowed to arbitrarily write and execute code is >12 months
My prediction: 70%. Resolution: hasn’t resolved yet (i.e., has not resolved to Incorrect yet)
While ChatGPT is able to run code (see image below), it isn’t allowed to execute arbitrary code, and in particular the ChatGPT plugins post notes that the Python interpreter is run “in a sandboxed, firewalled execution environment”.
I don’t know of any examples of AI chatbots that can execute code besides this one.
>24 months
My prediction: 50%. Resolution: Resolution: hasn’t resolved yet (i.e., has not resolved to Incorrect yet)
As above.
No publicly available product by the end of 2023 which is intended to make financial transactions (e.g. “buy an egg whisk” actually uses your credit card to buy the egg whisk, not just add it to your shopping cart)
My prediction: 90%. Resolution: Correct
ChatGPT refused to buy me an item (see screenshot below). The Copilot blog post has a “Shopping” section mentions finding what you want and finding the best price, but not actually purchasing it, so I think that supports my prediction.
For Google’s products, I couldn’t find anything about Gemini doing shopping or purchases, but Bard can’t make purchases.

Reflecting on these predictions
So of my 9 original predictions:
2 did not resolve
3/3 70% predictions were correct
2/3 90% predictions were correct
1/1 95% predictions were correct
Here’s what that looks like in the world’s-smallest-sample-size calibration chart:
This is a Brier score of .1575, and a log-score of .519.
From a calibration perspective, I think I made two mistakes:
I got a 90% prediction wrong. I went into the thought process that led to that prediction above, but on some level I think I just made the mistake of not phrasing my wording carefully enough. Namely, I framed my prediction as an “all” statement, while mentally treating it as a “most” statement. If I’m going to make such predictions, I need to consider the counterfactual far more carefully. All it took to prove me wrong was a single major company deciding to take a shot at a technically-feasible task, which I should have assigned more than a 10% probability to.
I was otherwise too cautious. Besides the above mistake, I hit every target, which means I was aiming too low. My natural inclination is to predict things that will happen, but then I should either give those things higher probabilities than 70%, or predict more things to bring my overall accuracy down to a 70%.
But although my calibration was imperfect, I’m very pleased with how well the original post holds up. This was published March 3, between the launch of Bing Chat and GPT-4. But I predict things like ChatGPT plugins (which were mostly “Quick Query tools” in the term I put there), the ease of multimodality (including the relative difficulty of video), and the challenges of translating LLM output to real-world actions.
Predictions are hard, especially about the future, and doubly-especially about the far far far distant future (9 months in AI). I somewhat regret that my predictions were on such a small subset of AI topics, but I think that was what let me get so many predictions right.